Employing a data mining approach for identification of mobile opinion leaders and their content usage patterns in large telecommunications datasets
Abstract
Recent technological advances in communication infrastructure, especially those in the realm of mobile services, have drastically impacted how people interact socially. The more ubiquitous and convenient mobile approach has steered away users from the traditional computer-mediated systems. As the mobile trend continues, un- derstanding usage behavior patterns of its users becomes critical to harness the power of this new technology. One particular user group, opinion leaders deemed tremendously influential on the usage behavior of other users, is the main focus of this study. Existing literature on the mobile usage pattern of opinion leaders is limited to theoretical rather than empirical studies. This research attempts to bridge the gap. Drawing from one of the largest Taiwanese telecommunications databases, we try to identify mobile opinion leaders and further cluster their mobile usage patterns by mining the actual data. This study exploits a combination of techniques, including statistics, data mining, and pattern recognition, in our data analysis. Four main characteristics and seven usage patterns of mobile opinion leaders were identified. There are two main contributions of this work. The first contribution is the application of opinion leadership theories applied in the traditional marketplace into mobile services based on a big data system; the second offers a taxonomy to logically analyze each pattern of mobile content usage behavior gathered from mining the data to provide better planning blueprint for future mobile resource consumption.
Introduction
The mobile services industry is growing rapidly, and more people are now adopting new mobile Web services. According to the reports of the International Telecommunication Union (Sanou, 2015), there were > 7 billion mobile cellular subscriptions worldwide in 2015, up from < 1 billion in 2000. Globally, 3.2 billion people are using the Internet and 2 billion of these users are from the developing countries. Further, a huge amount of data is increasingly being placed at the forefront of this communication due to the explosion of data coming from a wide array of technologies and greater/explosive Web content consumption (Chen, 2016; Garth, 2014). As the evolution of the mobile services industry proceeds even further, more value can be extracted by understanding the usage patterns of individual consumers or specific groups.
Studying specific groups and their influence from this gigantic and complex database, particular mobile Web services, is an important topic that has attracted the attention of a variety of marketing researchers.
Traditionally, people seek the opinion and advice of their peers re- garding various decisions they make. This behavior gives rise to a certain set of individuals in social networks, referred to by many as opinion leaders (Sharara et al., 2011). Considering the importance of these specific people or groups in social networks, previous studies have shown they have various titles (e.g., opinion leaders, key players and influential people; to be consistent, we suggest calling them as “opinion leaders”). In this paper, we use that term to refer to any influential group in the traditional social networks (Momtaz et al., 2011). From a marketer perspective, the early work that targeted women’s clothing fashion opinion leaders in media planning by Eric Vernette (2004) concluded that “the media planner can benefit from choosing opinion leaders as a specific media target because this will indirectly secure a free audience and can boost the impact of the advertising message” (p. 105). In this regard, opinion leaders potentially spread their messages through word-of-mouth to affect other users’ behavior.
Today, mobile services not only include voice and text messaging, but also provide computer-like applications, such as document viewers, Internet browsing, and multimedia for consumers to use and commu- nicate with other users. In addition, social changes have been un- precedentedly quick, and individual communications with others has become more frequent through the use of the mobile social networks (Turkle, 2015). Those mobile user patterns are more complex than those of the previous computer-mediated systems. In this way, the ex- ponential growth of mobile Web services provides opinion leaders with a wide range of social influence.
Meanwhile, marketers have always tried to develop communication strategies to meet the accuracy needs of mobile Web service content among the opinion leaders who informally influence the attitudes or behavior of other common people through product- or service-related conversation, referred to as “electronic word-of-mouth” communication. The study by Lu, Tseng, and Yu (2011) further suggested that mining usage patterns can help the discovery of specific (sub)groups, then used in applications like targeted specific favorite advertising and/ or personalization of content. Presumptively, mining Web content usage patterns can analyze the most suitable information to offer and serve as content preference mechanism to meet the needs of opinion leaders based on their actual mobile behavior (Lu and Tseng, 2009).
Surprisingly, little empirical research has yet investigated how mobile opinion leaders actually use Web content drawn from an amount of data analytics to further build up their usage patterns from data mining. Much of the contemporary writing on opinion leaders’ usage patterns of Web content consumption is theoretical rather than being empirically informed. Therefore, there is a need to move beyond the more generalized discussions on the characteristics of mobile opi- nion leaders and their usage patterns for Web content consumption in a specific developing country (e.g., Taiwan, holding one of the worl- d‑leading positions in technology and innovation, according to the Ministry of Economic Affairs’ reports in 2016) to look for rich empirical detail in the complex ways in which new technologies are being used in real ways and in the real world (Graham, 2004). Particularly, three main telecommunications entities in Taiwan hold a majority of the market shares, namely Chunghwa Telecom (35.4%), Taiwan Mobile (28.8%), FarEastone (28.6%) and others (7.3%) according to the 2015 shareholders’ meeting and report on FarEastone (2015). The data for this current study comes from one of these three telecommunication companies. One-third of all telecommunication usage market shares are in Taiwan, and they may provide phenomena to use and identify mobile opinion leaders and their Web content usage patterns. Based on the foregoing logic and suggestions, this study draws from one of the largest Taiwanese telecommunications databases to identify mobile opinion leaders and further cluster their Web content usage patterns by mining actual usage data.
The remainder of this paper is organized into five sections. First, the theoretical background of traditional opinion leadership characteristics discussion for identifying and examining hypotheses in mobile service data and mining user behavior patterns in mobile Web environments is described. Following that theoretical background, the research methods are explained. The final three sections discuss the results of the data analysis, the conclusions, and the managerial implications of the findings for theory and practice, as well as limitations and future research.
Theoretical background
Opinion leadership characteristics
Lazarsfeld et al. (1944) were the initial scholars who found that public communication does not directly flow to the mass, but is inter- preted first by opinion leaders and then delivered to the common people. The process was elaborated by Katz and Lazarsfeld (1955) and Katz (1957): the mass medium → the opinion leaders → the common people. That is the so-named “two-step flow of communication”. The main contributions of this theory are that (1) Information can be transferred not only by medium, but also by an interpersonal communication social network; (2) There is an interface between that medium and the interpersonal communication social network. More importantly, the interface is the opinion leader; (3) The influences of opinion leaders and interpersonal communication social networks on information communication and individuals’ decisions are far larger than that of any typical mass medium (Zhang and Dong, 2008).
Before making decisions, consumers often seek to reinforce their opinions through gaining consensual validation from certain others. Among these certain others are consumers who can exert an unequal amount of influence on the decisions of others; they are ‘opinion leaders’ (Rogers and Cartano, 1962). Opinion leadership refers to “the degree to which an individual is able to influence other individuals’ attitudes or behavior informally in a desired way with relative fre- quency” (Rogers, 2003, p. 271). In this light, opinion leaders are those consumers who influence the motivations, attitudes, opinions, beliefs and behaviors of others (Valente and Pumpuang, 2007). Although their influence is informal, opinion leaders do play an important role in the information acquisition of the consumer decision-making process and consequently can influence the outcomes of many marketing strategies (Assael, 1992). Indeed, Berkman and Gilson (1986) suggested that consumers believe the information they receive from communication with domain-specific opinion leaders to be more credible than that they receive from advertising messages.
While several theoretical frameworks for the characteristics of opi- nion leaders (Goldsmith and Flynn, 1994; Rogers, 2003; Weimann et al., 2007) have been proposed in traditional markets, Rogers (2003, p.316–318) has remained a popular theoretical model. According to his framework, the six generalizable characteristics of opinion leaders are:
(1) have greater exposure to mass media; (2) are more cosmopolitan;
(3) have greater contact with change agents; (4) have greater social participation; (5) enjoy a higher socioeconomic status; and (6) are more innovative. However, the work done on identifying opinion leaders by Chen and Weng (2015) proposed that current researchers have begun to notice new technologies and areas (e.g., mobile Web services) that have been largely ignored in the past.
With the expansion of mobile services, their work examined and suggested four main characteristics of opinion leaders that need to be considered to identify them from the mobile service data. In this study, the task of determining characteristics for identifying mobile opinion leaders utilized the work of Chen and Weng (2015), mainly including enduring involvement, exploratory behavior, innovation, and mobile competence. Following the descriptions given each characteristic in terms of appropriateness from a theoretical perspective, these four characteristics were used to identify and examine the hypotheses re- garding mobile opinion leaders as drawn from the telecommunications database.
Enduring involvement
Involvement is the interest, enthusiasm, and excitement that con- sumers feel for specific product/service categories, and it reflects the personal relevance of these categories. Given its widely accepted the- oretical framework, consumer involvement can be distinguished be- tween enduring involvement as an individual attribute and situational involvement related to a specific behavior (Bergadaa and Faure, 1995). According to these concepts, enduring involvement emphasizes long- term attachments rather than transitory situational feelings or states (Jang and Lee, 2000). Prior studies have suggested that enduring pro- duct/service involvement is one of the major underlying motives of opinion leaders, whereas situational involvement bears no relationship to opinion leadership (Richins and Root-Shaffer, 1988; Venkatraman, 1990).
Consumers with enduring involvement will demonstrate an interest and familiarity with a product/service over time (Valente and Pumpuang, 2007). Consumers will maintain perceptual vigilance for information concerning products/services with high enduring im- portance and maintain a strong and continuing interest in that product/service for its own sake (Bloch et al., 1983). Enduring involvement motivates consumers to acquire knowledge and share information about product/service categories in which they have developed high levels of expertise (Lyons and Henderson, 2005). Allen (2001) identi- fied enduring involvement as a factor that contributes to opinion lea- dership. Given that the mobile service presents opinion leaders with an unprecedented repository of information, we used the first character- istic as those for an enduring involvement to identify mobile opinion leadership and examine the hypotheses from our mobile database (Chen and Weng, 2015). First, we hypothesize that:
H1. Mobile opinion leaders have higher levels of enduring involvement with mobile services than non-opinion leaders do.
Exploratory behavior
Opinion leaders engage in exploratory behavior to attain a sa- tisfactory level of stimulation (Raju, 1980). Regardless of whether they are motivated by curiosity, basically a need to try something different, their goal is to gather previously unknown information. In general, opinion leaders who demonstrate high levels of exploratory behavior seek out new, surprising, and intense activities. They are also likely to be among the early adopters of products, services, and ideas. In this light, opinion leaders with greater exploratory behavior tendencies are likely to be more aware of developments in areas of their own interest and display a greater tendency to evaluate, try, accept, and adopt new innovations (Goldsmith and Desborde, 1991).
Earlier studies conducted in computer-mediated environments support the exploratory behavior theories that have been developed in traditional markets. Opinion leaders who seek higher levels of stimu- lation within a computer-mediated environment will exhibit more ex- ploratory behavior (Lyons and Henderson, 2005). These increased le- vels of exploratory behavior lead to enjoyment, as opinion leaders adjust to the challenges of the mobile service environment where more Web services are provided today. It will be assumed that opinion lea- ders can derive pleasure simply from the act of exploring these mobile service environments. Exploratory behavior was considered to be the second characteristic for identification in this study (Chen and Weng, 2015). Sequentially, we then hypothesize that:
H2. Mobile opinion leaders possess higher levels of curiosity and exploratory behavior with mobile services than non-opinion leaders do.
Innovation
Innovativeness is a measure of a consumer’s willingness to adopt a new product/service without seeking the advice of others. Since opinion leaders seek to create and maintain meaningful social relation- ships, they often engage in behavior approved by others, such as adopting a new product/service so as to appeal to other product/service adopters (Cialdini and Goldstein, 2004). Referring to the degree of innovativeness of such opinion leadership, Lyons and Henderson (2005) elaborated, saying “Compared with consumers who seek their advice, opinion leaders frequently possess more experience or expertise with the product [/service] category, have been exposed to or acquired more information about the product [/service], exhibit more exploratory and innovative behavior, and display higher levels of involvement with the product [/service] category” (p. 320). In this light, opinion leaders possess high levels of innovativeness, and the advice they provide to other consumers strongly influences consumption behavior (Hirschman, 1980). Opinion leaders are often new product/service adopters who transmit product/service information and their own usage experience to other people. Accordingly, innovation was seen as being third characteristic for identifying mobile opinion leaders from our mobile database (Chen and Weng, 2015). Thus, it is hypothesized that:
H3. Mobile opinion leaders have higher levels of innovativeness with mobile services than non-opinion leaders do.
Mobile competence
In traditional markets, opinion leaders use a variety of skills, in- cluding reading, listening, and watching/observing, to acquire high levels of product/service packaging, develop enduring involvement with a product/service category, and become innovative product/ser- vice adopters. These attributes are characteristics of opinion leaders. With the advent of computer-mediated environments, computer com- petence reflects the requisite computer skills that enable consumers to navigate the Internet successfully and cope with the demands imposed by computer-mediated environments (Hoffman and Novak, 1997). Consumers must develop at least a minimal level of computer compe- tence before they can access the Internet; thus other persons with limited competence are constrained from maximizing their usage of the information capacity of the Internet. Some studies have found that computer competence enhances individual performance and pro- ductivity (Webster and Martocchio, 1992). Hoffman and Novak (1997) further suggested that high levels of computer skills result in increased confidence levels. As consumers acquire more skills and attain a degree of competence, they become more comfortable with playfully experi- menting with their computer use (Webster and Martocchio, 1992). In other words, higher levels of computer skills result in increased in- volvement and greater satisfaction levels. Indeed, the work of Lyons and Henderson (2005) highlighted that opinion leaders who are more skillful with computers are likely to explore the Internet more thoroughly.
Accordingly, opinion leaders who possess high levels of mobile competence will demonstrate increased confidence that leads them to experience greater satisfaction and involvement with mobile services (Chen and Weng, 2015). They display high levels of exploratory be- havior and spend longer periods of time on mobile service systems. Based on the suggestions of Chen and Weng (2015), mobile competence was considered to be the fourth characteristic to use for identifying mobile opinion leaders from our mobile database. Finally, we further hypothesize that:
H4. Mobile opinion leaders possess higher levels of mobile competence and spend longer periods of time on mobile services than non-opinion leaders do.
In this study, we did not just identify mobile opinion leaders and examine our hypotheses from reviewing the quantity and variety of data. We also tried to gain greater insights and more knowledge about the Web content usage patterns of mobile opinion leaders from mining the data analytics. To do so, we reviewed the following literature on mining user behavior patterns in mobile Web environments to build up the foundations for research methods and data analyses still further.
Mining user behavior patterns in mobile web environments
Today, young consumers are growing up in a media-rich environ- ment. Their use ranges from the more traditional ways (e.g., listening to music) to the more interactive and ubiquitous services (e.g., surfing the Internet, watching video and audio films, playing multi-digital games, sharing information on Facebook, shopping, etc.) available on mobile devices. The concept of interactive and ubiquitous computing in the mobile domain is used when referring to today’s heterogeneous mobile services. It also refers to a trend wherein mobile services integrate with the Web environment, improving its content values through en- tertainment, information, e-commerce, or social networking. Although marketers understand mining user patterns based on content-interest driven behavior is becoming more important, it is well known that finding behavior patterns from a huge amount of data is a stereo- typically expensive and difficult task.
In recent years, more and more scholars have becoming involved in data mining as a popular and more widely used technique for dis- covering valuable information and usage patterns from gigantic and complex data (Han and Kamber, 2000; Lu, Lee, and Tseng, 2011). For instance, Tseng and Lin (2005) first proposed finding consumers’ mobile access patterns. Wang and Cheng (2011) used a sampling approach to find the periodic maximal promising movement patterns of mobile phone users. The results showed that users’ popular services are totally different in various time periods. Further, Lee et al. (2007) proposed finding temporal mobile access patterns for different time intervals. Their results suggested that although users’ mobile access patterns are vital, their moving paths are also essential. For example, if a girl arrives at university area, administration could recommend her useful in- formation at the university based on most of students being used the service information at current time. She would move to nearby opened library for checking out books.
Generally speaking, the results of prior studies are both helpful and useful in the academic field, but most have focused on mining common consumer behavior patterns. From the academic and managerial per- spective, we argue that this focus is less beneficial than the present paper, which emphasizes the mining of mobile opinion leaders. Because mobile opinion leaders are seen as influential groups, understanding their actual Web content usage patterns can help to precisely analyze each pattern of market shares on the basis of Web content-interest consumptions. Further, these interest messages can potentially diffuse to other users through an effective marketing (e.g., advertisement sharing) strategy. In order to gain the precision behavior of mobile opinion leaders, we need an effective personalization of the mobile behavior pattern mining approach. The personalization of Web content usage pattern mining systems can analyze the most suitable information for mobile opinion leaders based on their individual Web content-in- terest consumption. Once the clustering patterns of mobile opinion leader subgroups in Web service environments can be determined, their Web content-interest preferences can be simultaneously indicated. More essentially, marketers can then precisely send recommended messages and resources through their advertising or promotion activity to satisfy their needs and goals (Lu and Tseng, 2009; Lu, Tseng, and Yu, 2011). Furthermore, in the more traditional analysis of user behavior patterns, consumers are usually clustered by their personal profiles, such as demographic variables, including age, sex, and income. In the current research, we tried to overcome these existing obstacles and transmuted the effort from more traditional simple data profiles to the personalization of the mobile Web content usage pattern mining ap- proach. More details on these research methods are described as the following section.
Methods and data
Theoretically, selection of the most appropriate methods for a re- search effort depends on the setting, availability of appropriate opinion leaders, and available resources. It is also possible that the techniques used may depend on the role expectations of mobile opinion leaders in this study. For example, these techniques are expected to identify mo- bile opinion leaders and examine hypotheses using the quantity and variety of data and further release their usage behavior patterns, such that suitable Web content usages can be analyzed (Tseng and Lin, 2006; Vernette, 2004). In recent years, new techniques and technologies have been introduced, ranging from manipulating and visualizing to ana- lyzing big data. The techniques associated with big data analytics in- volve a number of disciplines, including statistics, data mining, ma- chine learning, neural networks, social network analysis, pattern recognition, optimization methods, and visualization approaches (Chen and Zhang, 2014; Ishwarappa, 2015). Based on these concepts, this study exploited a combination of the techniques for the entire research project, including statistics, data mining, and pattern recognition. There are two stages of timeline research that can identify mobile opinion leaders and also examine our four hypotheses based on literature reviews in the first stage, as well as continuously mining and clustering their Web content usage patterns in the second stage.
In the first stage of this timeline research, the data were drawn from the aforementioned Taiwanese telecommunications company from April 2014 to September 2014. Basically, this telecommunications company randomly provided us with 66,578 counts1 of mobile users, including accuracy of users’ personal profiles. For each user, it further collected the duration record and fundamental mobile phone usage information (e.g., receiving, calling and texting counts or mobile phone bills per month, etc.). In order to identify mobile opinion leaders more accurately, we set rules based on the previous literature that indicated that mobile opinion leadership must match all the criteria we have mentioned in the theoretical framework, including enduring involve- ment, exploratory behavior, innovation, and mobile competence (Chen and Weng, 2015). First, according to the available resources provided from this telecommunications access, for enduring involvement, te- nure2 is appropriate as are the states for the active months since the users joined the telecommunications services.
Next comes exploratory behavior, and CWC3 index refers to whether users are willing to contact the telecommunications consumer service center. In other words, these users not only check their billing status, but also consult for an unlimited data plan and/or mobile promotion information. Unlimited data allow users to do their Internet browsing more easily and likely also adopt unlimited services effectively. That is, adopters whose willingness of information research for these unlimited data can be higher than that for limited data packaging users. Third is innovativeness. We categorized the high-end mobile phone brand and device as the standard. In the current technological era, Apple, Sam- sung, HTC, LG, and Sony mobile phone brands and multi-functional devices are used more extensively in Asia. Moreover, GPRS4 usage is described as general packet radio service with widespread of 4G In- ternet usage, and the mobile device must adopt LTE (Long-Term Evo- lution. It is a standard for high-speed wireless communication for mo- bile phones and data terminals) in order to apply for 4G services. Therefore, the innovative mobile devices are also taken into account. Fourth, mobile competence consists of data usage, calling and receiving usage and SMS (Short Message Service) usage. This variable contains users’ real time usage behavior, including both Web contents/websites and traditional calling services. The frequency of usage indicates the calling and receiving counts of mobile services as well as the surfing of Web contents.
Overall, we determined that mobile opinion leadership may conform to all of the variables above and the accumulation of usages must be in the top 30% of each variable, according to past studies that have split the sample for 20% to 30% of respondents and designated them as ‘opinion leaders’ (Goldsmith and Flynn, 1994; Lyons and Henderson, 2005). In the first stage, we exploited statistics to test the hypotheses and identify mobile opinion leaders. It became clear to us that big data research approaches themselves do not create new knowledge. Rather, they are just tools that when used appropriately on carefully collected and analyzed data, theory and knowledge can then be generated.
In the second stage of timeline research, this paper takes further Web content usage patterns into consideration; thus the techniques of data mining and pattern recognition were adopted. Today, mobile services can assist for a variety of contents, such as going shopping, navigating to a particular point of interest, retrieving online news, and purchasing airline tickets. As mentioned in the theoretical background section, understanding or mining the personalization of content-interest driven value approach is not unique. Ranto et al. (2005) have devel- oped tools for identifying context or content. They identified clusters of locations and contexts and provided artificial intelligence when making guesses on users’ contexts or content and presence information. Con- sulted on their work and available resources in this study, we adopted the mining of the personalization of content-interest driven approach at the second stage (Raento, et al., 2005). Logically based on the results of the first stage which identified 1161 mobile opinion leaders, we asked for further mining based on their actual utilization of Web content usage data. The raw data, including all of the mobile opinion leaders’ actions with the Smartphone over a panel project of a month (from December 1st to 31) in 2014, were used together with accurate time interval consumption when determining all kinds of Web content usage. In data mining, clustering produces a grouping of people and fore- casting is a key task. As certain authors suggested (Zliobaite et al., 2016), forecasting applications typically relates to analytics tasks in marketing, such as demand prediction, consuming time predictions, and event prediction. Applying these suggestions, we clustered each pattern by usage-level differences between content interests/pre- ferences and time intervals. Regarding the setting of time intervals for this research from the mining of data, the rules divided a day into four equal parts of a 24-h day. Midnight was set from 00:01 a.m. to 6:00 a.m.; morning was fixed from 6:01 a.m. to 12:00 a.m.; afternoon was ordered from 12:01 p.m. to 18:00 p.m.; and night was regulated from 18:01 p.m. to 24:00 p.m. All in all, 658,276 counts of data were mined using these criteria from 1161 mobile opinion leaders. To pre- cisely outline each pattern of Web content usage for mobile opinion leaders, cluster analysis was applied to the data. Because there was no a priori classification scheme, a hierarchical agglomerative cluster ana- lysis was applied instead of using discriminant or assignment methods (Raento et al., 2005). Once both the factor analysis and the exploratory cluster analysis were applied and resulted in categories, there was no need for additional variance analyses. It has been suggested that mobile opinion leader subgroups whose Web content interest paths and time intervals are similar can be clustered into the same category (Lu and Tseng, 2009; Lu, Tseng, and Yu, 2011).
Results and analysis
Identifying mobile opinion leaders
Drawing from a huge amount of data from the telecommunications database, opinion leadership showed roughly at 1.78% or totally 1161 users (65,097 users *0.0178) who were potential mobile opinion lea- ders (Momtaz et al., 2011; Sharara et al., 2011). Previous studies have partitioned the samples to correspond as closely as possible to the 20% to 30% of the population already identified as opinion leaders in the extant marketing literature (Koter et al., 1994; Goldsmith and Flynn, 1994; Lyons and Henderson, 2005). The outcomes of this study are consistent with the opinion leader theory. It is important to notice that this study not only provides a theoretical framework to identify mobile opinion leaders, but it also logically and systematically partitioned its sample into “opinion leader” and “non-opinion leader” categories.
The detailed profiles of mobile opinion leaders showed as follows: Table 1 reveals slightly more females (51.6%) than males (48.4%). The age of mobile opinion leaders mostly falls into main two ranges be- tween 26–30 (22.2%) and 31–35 (28.7%) years old. In addition, most mobile opinion leaders are located in Northern Taiwan (52.5%).
In the first stage, the purpose of this study was to identify whether the main four characteristics of mobile opinion leaders as derived from the telecommunications dataset are similar to the characteristics of opinion leaders in traditional markets. Moreover, the hypotheses were devised as predicting that positive relationships exist between mobile opinion leaders and non-opinion leaders within the mobile service marketplace. Table 2 provides a summary of the hypotheses and the results of the hypothesis variables obtained in this study. The analysis indicated that the mean scores and t-test comparisons between mobile opinion leaders and non-opinion leaders across the entire dataset were statistically different (ρ = 0.000). A MANOVA was performed on two groups of mobile opinion leaders and non-opinion leaders as the in- dependent variables and on four characteristics as dependent variables. The Box’s M test indicated that the homogeneity assumption was valid (Box’s M = 4868.24, ρ = 0.000). Levene’s test of equality of error var- iance showed no evidence of unequal variances. Wilks’s Lambda was used to assess any multivariate differences across the groups. This analysis showed that the mean scores on all four of the dependent variables were statistically different (F = 881.053; df = 5; ρ < 0.001).
Generally speaking, the directions of these differences for each characteristic were all consistent with the hypothesized relationships found in prior studies (Chen and Weng, 2015; Lyons and Henderson, 2005). These results supported the hypotheses that mobile opinion leaders demonstrate greater involvement levels with mobile services or the Internet and possess higher levels of mobile competences with mobile services than do non-opinion leaders. Furthermore, this study found that mobile opinion leaders possess higher levels of innovative adoption of mobile new brand types (e.g., Apple, Samsung, HTC, LG, Sony, etc.) and 4 G services to browse the Internet as well as demonstrating higher levels of curiosity and exploratory behaviors than non-opinion leaders do. These mobile opinion leaders logged onto the Internet by mobile devices often, and they are likely to surf the Internet using mobile de- vices to see what is there and also spend more time on exploring a diversity of Web contents/websites.
Table 3 further shows the top twenty Web content usages based on the exploratory behavior of mobile opinion leaders using data analytics. The former top ten of exploratory behavior is described as follows: The first ranking of Web-related content usages is the search engine, namely, Google. The second and third rankings of Web content usages are video-and-audio sharing plus search engine functions, respectively, Google videos and YouTube. The fourth ranking is one of the Taiwanese Web forums called Mobile 01 wherein people get together to share a variety of products or services information and recommend ideas to others. The fifth and sixth rankings are social networking sites, re- spectively, Facebook and Instagram. The seventh and eighth rankings are Taiwanese famous online news outlets, respectively, Ettoday News and Apple Daily News. The ninth ranking is a popular game for young adults, namely, Candy Crush. The tenth ranking of Web content usage is a Chinese instant chat, namely, QQ.
The rests of the latter rankings within twenty exploratory Web content usages mostly belonged to the functions of search engine, gaming, audio and video entertainment, daily life tips searching, gambling, porn movies/videos, and e-commerce. In order to understand the deeper insights of exploratory behavior patterns among mobile opinion leaders, we suggested that the telecommunications company provide wider and deeper mining data on Web content consumptions among mobile opinion leaders to analyze their actual usage behavior patterns at the second stage. The analytics of Web content usage pat- terns among mobile opinion leaders were as follows in Table 3:
Analytics of web content usage patterns for mobile opinion leaders
The original dataset included 1161 mobile opinion leaders who were identified in the first stage. After mining the Web content usage algorithm for the entire dataset, there were totally 658,276 counts and 412 mobile opinion leaders who were active in that month and to whom the Web content usage algorithm accurately identified separate categories in the second stage.
In mining data analytics, this study took usage terms from logs of Web contents of mobile opinion leaders’ actual exploratory behaviors and organized them into categories. Originally, the Web contents were labeled as diverse websites, resulting in thousands of different labels. To do that, we first decreased the number of labels. Sequentially, we la- beled each with similar Web contents as a specific category based on core activity and also as consulted by previous studies (van den Beemt et al., 2011). As a result, the main eight categories were identified using the above suggestions. One content category consisted of searching for information and surfing the Web, such as Google, Baidu, Yahoo, etc. Because these are traditional computer-mediated systems, more basic interactive Web media activities focused on the non-linear consumption of information and the production of texts. We labeled this category as “information searching”. A second content was called service-needed driven and consists of a variety of service orientations (e.g., software service provider, daily life tips, psychological and physical needed re- sources, etc.). We identified this category as “e-service exploring”.
A third content, consisting of joining to look for partnerships or intimate relationship activities, is a form of making friend- driven or joining Web dating activities where users look for dating chats or sites for fun through Web services. We named this category as “e-dating”. A fourth content is called friendship manage- ment driven (Ito et al., 2008), and consists of a variety of social networking activities (e.g., Facebook, Instagram, mobile 01 forum dis- cussion, etc.). We labeled this category as “social networking”. A fifth content, consisting of multi-gaming activities (e.g., Candy crush, gam- bling games, etc.), is a participation driven or so-called role performing where users play a certain role on mobile devices (Ito et al., 2008). We named this category as “e-gaming” or “e-performing” (Duimel and De Hann, 2007). A sixth content, consisting of participating e-commerce, e-consumption or e-promotion activities, is a form of joining e-shopping events or activities where users are keen to purchase products/brands through using e-commerce services. We identified this category as “e-shopping”. A seventh content can be called e-news reading driven, and consists of getting newly received or noteworthy information (e.g., Ettoday, Apple Daily News, etc.), especially on recent or important events through mobile Web services. We called this category as “e-news reading” (Livgstone and Bober, 2005). The last content is video-and-audio viewing driven and consists of a variety of viewing materials (e.g., Korean drama, animations or movies, documentaries, etc.) de- livered through mobile Web services. We labeled this category as “e-video viewing”.
Fig. 1 presents the average four-time intervals distribution of the main eight aforementioned categories among the mobile opinion lea- ders who engaged in utilizing Web content consumptions within a month from the data mining. Based on these aggregate data, the rankings and ratios of these eight categories are: Information searching (51.5%), social networking (14.6%), e-video viewing (9.2%), e-service exploring (8.9%), e-shopping (5.4%), e-news reading (5.3%), e-gaming (3.9%) and e-dating (1.2%). It also shows that mobile opinion leaders who engaged in “information searching” had the highest frequency (240,857 times) of Web content usage among these eight categories, and active time intervals, which occurred from afternoon (67,106 times; 27.8%) to night (78,038 times; 32.4%). In information searching, the ratios of gender showed that females (53.8%) are slightly higher than males (46.2%); the majority of age was located in two ranges between 30 and 40 (49.9%) and 20 to 30 (30.7%), and the average age was 32.7 years old; and the majority of living locations were Northern (45.4%) and Southern (35.5%) Taiwan. All in all, the ratios of gender, age, and living location among the rest of the categories were similar to those for information searching. Beside “e-shopping”, there are largely females (60.5%) more than males (39.5%) engage in e-commerce ac- tivities using mobile devices. In contrast, men (57.8%) outnumber women (42.2%) in joining “e-dating” activities on mobile devices, even though that usage database is not that big compared to the other seven categories in this study.
The important difference is active time interval allocation among the eight categories. Beside “e-service exploring”, the time intervals of it are similar to those of information searching from afternoon (31.3%) to night (30.3%). The active time intervals of both “social networking” and “e-news reading” cross three zones from morning (24.7%; 20.7%), afternoon (29.7%; 33.6%) to night (26%; 28.5%), respectively.
Similarly, the peak of both categories is mainly in the afternoon. Profoundly, the prime time of mobile opinion leaders who engage in “e- shopping” is mainly located at night (41.2%) from 18 p.m. to midnight. Interestingly, the time intervals for “e-video viewing” evenly cross four time intervals (midnight 21.3%; morning 24.2%; afternoon 25.9%; night 28.5%); that is, they are almost active for 24 h. As expected, the prime time intervals for mobile opinion leaders who participated in “e- gaming” activities were located at night (37.2%) and midnight (27.4%). Mobile opinion leaders who join “e-dating” events were fewer, and the time intervals of their engagements were mostly located at night (31.7%).
It is essential to cluster specific patterns from these aforementioned eight categories, particularly in terms of centrality, connectivity, and subgroup structure to categorize Web content usage behavior for spe- cific interests or lifestyles of mobile opinion leaders (Totten et al., 2005). Generally speaking, it is clear that the interest of each category has an underlying structure, and perhaps we ought to consider grouping the patterns based on shared categories’ usage. The dataset is quite extensive, but we first take centrality into account based on the char- acteristics of mobile opinion leaders from previous studies (Chen and Weng, 2015; Goldsmith and Desborde, 1991; Lyons and Henderson, 2005) and similar time intervals spent consuming from those afore- mentioned results. As the previous literature has mentioned, opinion leaders express characteristics that are keen to explore new information and/or relevant services (Lyons and Henderson, 2005). More im- portantly, they are keen to share and spread those messages to others (Vernette, 2004). Accordingly, the centrality of the three categories for the characteristics of mobile opinion leaders in Web content con- sumptions are information searching, e-service exploring, and social networking.
Based on these three foundation categories that are directly associated with the characteristics of mobile opinion leadership, we further clustered the Web content usage patterns for the rest of the categories. We also looked for stable connectivity and a subgroup structure that in the data (Totten et al., 2005). In the clustering process, it was noticed that some specific categories stably linked into a single subgroup. For instance, information searching, e-service exploring, social networking, and e-shopping clustered together as a pattern that was labeled as “e- shopper”. Another subgroup pattern came from information searching, e-service exploring, social networking, e-video viewing, and e-shopping clustered together as another pattern named “e-shopping and e-video driven enjoyer”. As a result, seven main patterns of mobile opinion leader subgroups were clustered, and they included e-news browser, e-video viewer, e-shopper, e-news and e-video driven viewer, e-shopping and e-video driven enjoyer, e-news and e-shopping driven action-taker, and all-service user.
Sequentially, we exploited the Venn diagram to explain the clus- tered results that the main seven Web content usage patterns of mobile opinion leader subgroups have as a specific interest that emerged from the telecommunications mined data (Chan et al., 2011). Fig. 2 shows the Venn diagram visually with all the possible logical relations for each pattern. The ratios of each pattern and the main time intervals of its core activities were as follows:
- e-news browser (1.11%) takes place mainly at night;
- e-video viewer (6.64%) falls out mostly in the afternoon;
- e-shopper (1.85%) happens mostly in the afternoon;
- e-news and e-video driven viewer (28.41%) and an overlapping peak time interval of core activities occurring mainly in the morning;
- e-shopping and e-video driven enjoyer (11.07%) and the over- lapping peak time interval of its core activities coming profoundly at night;
- e-news and shopping driven action-taker (3.69%) and overlapping peak time interval of its core activities mainly in the afternoon;
- all-services user (47.23%) and overlapping peak time interval of its core activities extremely at
The basic profiles for each pattern are shown in Table 4. Interest- ingly, except for a pure e-shopper, for which the ratio of usage is small, each pattern associated with the category of e-shopping highlighted that females (67% of e-shopping and e-video driven enjoyer; 60% of e- news and e-shopping driven action-taker; 59.4% of all-services user) outnumbered males. In contrast, beside e-news and e-shopping driven action-taker, each pattern related to the categories of e-new viewing and e-video viewing releases where male viewing (e.g., 100% of e-news browsers; 66%of e-new viewers; 51%of e-news and e-video driven viewers) was slightly larger than females. The average age of those in each pattern was 33.3 years old. The locations of each pattern fell into Northern and Southern Taiwan.
According to these determined results, marketers can gain knowl- edge of each pattern behavior. Each pattern of mobile opinion leader subgroups (e.g., e-shopper, e-video viewer, etc.) has its own lifestyle and routine activities based on interests and time-intervals for con- suming Web content. It is an excellent way for marketers to provide accuracy value and deliver relevant messages in precise timeframes to attract a specific lifestyle of different mobile opinion leader subgroups for marketing strategies (Michman and Mazze, 2009). More im- portantly, the Venn diagram in this study further evaluates the beha- viors and market shares of logical relationship patterns. In other words, this diagram can be interpreted as the relationships of patterns. We use two patterns such as e-shopper and e-video viewer, for example, to il- lustrate how these two mobile opinion leader subgroups can evaluate the size of its logical relationship markets (or potential market shares) and its behavior patterns. In Figs. 2, 5.54% for left shape as e-shopper (1.85%) and e-news & shopping driven action-taker (3.69%) among mobile opinion leader subgroups whose core lifestyle tend to be more e- shopping and particularly spend time in the afternoon. On the other hand, 35.05% for right shape as e-video viewer (6.64%) and e-news & video driven viewer (28.41%) among mobile opinion leader subgroups whose core lifestyles are apt to be more e-video viewing orientation and mainly engage in the afternoon. Profoundly, marketers can accurately measure the market share of its lifestyles related to e-shopping with e-news browser or e-video viewer with e-news browser to send specific messages and relevant advertisements to them. Furthermore, Fig. 3 and Fig. 4 present the moving paths of both “e-shopping and e-viewing driven enjoyer” and “all-services user” in four time intervals. Marketers greatly increase the accuracy of evaluation that the overlapping peak time interval consumption of routine activities between e-shopping and e-viewing driven enjoyer and all-services user appears mostly at night.
Conclusions and managerial implications
Big data today is large, varied, and complex and can directly reflect society changes and behavior of individuals or specific groups (e.g., a mobile opinion leader in the current study). However, many theories, methodologies, technologies, and analytical techniques are still needed or must be upgraded to accurately extract the most relevant informa- tion. Overall, contributions of each stage of this current research have added to the theory and managerial implications of the research for actual marketing practice. They are as follows:
In the first stage, the purpose of this study was to examine whether the main four characteristics of opinion leaderships are appropriate for identifying mobile opinion leaders as derived from the tele- communications dataset. Of the four hypothesis variables, enduring involvement, exploratory behavior, innovativeness, and mobile com- petence were significant for distinguishing mobile opinion leaders from non-opinion leaders. General support was found for all of the hypotheses, thus lending support to the idea that identifying mobile opinion leaders from a huge dataset can be applied from the theoretical background. A key contribution of the first stage of this study applies opinion leadership theories developed in the traditional marketplace into a mobile services marketplace using the big data system, thereby supporting the appropriateness of a theoretical foundation that can be used to identify mobile opinion leadership.
In the managerial implications of the first stage, mobile opinion leaders are an ideal segment of early adopters for telecommunications companies to target with new service information because they are keen to share their knowledge with less-informed, less-innovative col- leagues or other mobile users through mobile services and social media. The information that mobile opinion leaders diffuse to colleagues and even countless online news forum groups (e.g., Mobile 01 in Taiwan) can alleviate apprehension and any lack of adoption confidence (e.g., 4G adoption) for other mobile users. Telecommunications companies should see mobile opinion leaders as potential mobile service cham- pions who can act as messengers to less-confident and less-knowl- edgeable users in the mobile marketplace.
Telecommunications companies can initiate the process of identifying mobile opinion leaders based on their own big data system. Their data collection should focus on mobile services (e.g., calling, receiving, texting, etc.) and their Internet usage history as well as their level of mobile competences and activities. Once mobile opinion leaders are identified through these aforementioned actions and routine activities, telecommunications companies can target that key segment of the Internet and mobile usage population with unique Web services and thus build long-term relationships. For example, companies could offer mobile opinion leaders electronic newsletters, and special e-shopping promotions about new services or products. These mobile opinion leaders are often keen to explore this new information and potentially spread informative messages through electronic word-of-mouth to affect other users.
Our key contribution for the second stage is the study of exploratory behavior of mobile opinion leaders. Such behavior is valuable for un- derstanding opinion leaders’ Web content usage patterns. Theoretically, lifestyles are defined and expressed through distinctive consumption patterns (Holt, 1997; Warde, 2005). Existing literature on lifestyle theory is limited to conceptual rather than empirical studies. In this study, we attempt to empirically study lifestyles through the proposing of seven key patterns among mobile opinion leader subgroups to logi- cally and systematically cluster different lifestyles. In doing so, we applied data mining and analysis to systematically measure users’ consumption patterns on the basis of their Web content and interest preferences and routine activities.
In terms of the managerial implications of the second stage, the results of clustering Web content usage patterns for this study echo what Gilad de Vries (2015), a Senior Vice President of Atrategy at Outbrain, claimed regarding Web content consumption (e.g., Vogue, GQ, Time e-magazine, CNN, etc.). It represents the “interest” of consumers (or mobile opinion leader subgroups in this study). It can become a popular trend for marketers to use to analyze Web content consumption behavior patterns of their target consumers using mobile devices. In other words, it provides unique and extremely accurate data sets to help marketers uncover the true interests of their target con- sumer or specific subgroup. For example, this study mentioned earlier that e-shopping and e-video viewing enjoyer performs best on mobile devices at night between 20 p.m. and midnight. A lot of this subgroup of mobile opinion leaders want to relax or entertain themselves and are very receptive to surfing or watching that kind of content during those times. Such behavior and routine activities are seen as lifestyles. Si- milarly, Giddens (1991) elaborated lifestyles as Lifestyle… implies choice within a plurality of possible options… is ‘adopted’… [and] routinized practices, the routines incorporated into habits of dress, eating, modes of acting and favored milieux for From managerial perspective, the lifestyle concept is one of the most widely used strategies in modern marketing. It provides a precise way to understand consumers’ everyday needs and wants. It also is a me- chanism that allows a product or service to be positioned in terms of how it will allow a specific type of person to pursue his or her desired lifestyle (Michman and Mazze, 2009). This study highlights that each pattern among mobile opinion leader subgroups indicates the routines incorporated into habits of Web contents they surf/browser and the times they spend when engaging in mobile services (de Vries, 2015; Michman and Mazze, 2009). These choices in turn create opportunities for market segmentation strategies that recognize the potency of each chosen lifestyle for determining types of specific services that are more likely to appeal to their designated lifestyle segment. Essentially, the logical relationship between each pattern may help marketers analyze that type of suitable content to consider for strategic alliances in mar- keting promotions.
Limitations and future research
We acknowledge that like any piece of research, this study has its own limitations. For example, applications usage data in this study are not fully included in the telecom dataset. The ownership of the appli- cations usage data belongs to the applications creator. Telecom Company randomly detects the Web content usages and the usages of calling and receiving data. Thus, some of the measurements are limited by the dataset and available resources. For example, one characteristic of mobile opinion leaders such as enduring involvement might be measured by how often users acquire knowledge and share information about product/service. In other word, it is difficult to detect such measurement under aforementioned criteria. From our perspective, if the database can expand to the diversity, we might enhance the mea- surement accurately. Similarly, innovativeness of the mobile user could stand for different aspects. In this study, one of the mobile innova- tiveness reveals in service usage. Users are willing to try the newest applications in order to experience varies service in applications such as food delivering service or personal taxi service. Yet, all of the actions based on the applications do not appear in telecoms’ dataset. Alternatively, we considered innovativeness of the measurement to be the user mobile device and data plan, which were available in our dataset. Because of the high demanding of the application download and searching behavior, hi-end or unlimited data plans would be the one of the possibility to describe the users.
For future study, lifestyle theory should include specific measures of (mobile) activities and personal characteristics (or demographic fac- tors) based on the review of lifestyles literature (Holt, 1997; Giddens, 1991; Warde, 2005). In addition, mobile opinion leaders potentially can influence both the strong tie of mobile social networks (e.g., relatives, close friends, etc.) to the global audience of unlimited users involved with mobile Web social media (e.g., Facebook, Instagram, etc.) and forums (Sharara et al., 2011). The results of this study do leave more issues to investigate for how marketers can develop precision commu- nication strategies to reach each pattern of mobile opinion leaders who also informally can influence the behavior of other mobile social users with whom they connect.
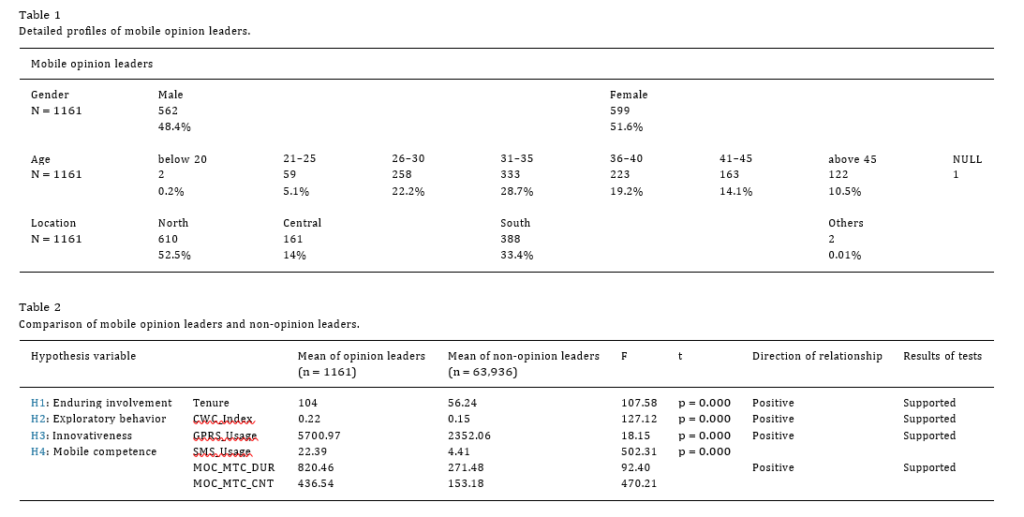
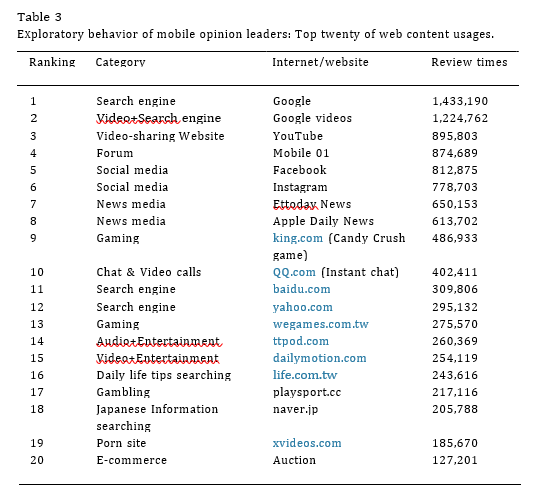
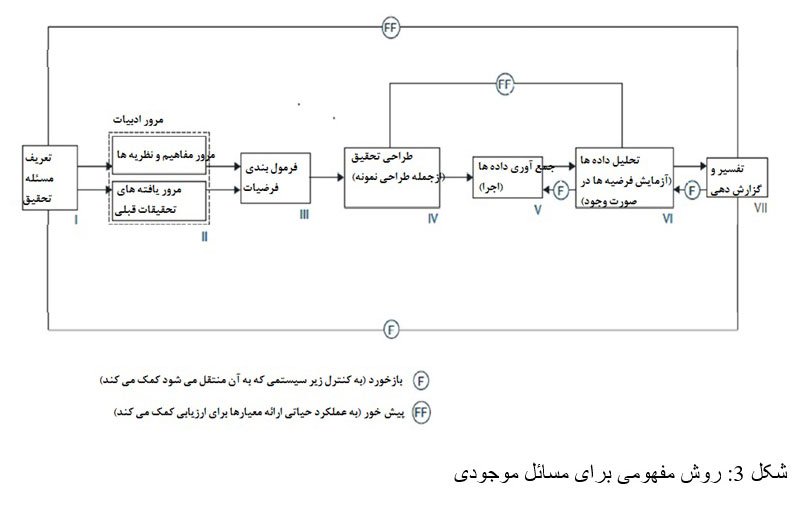
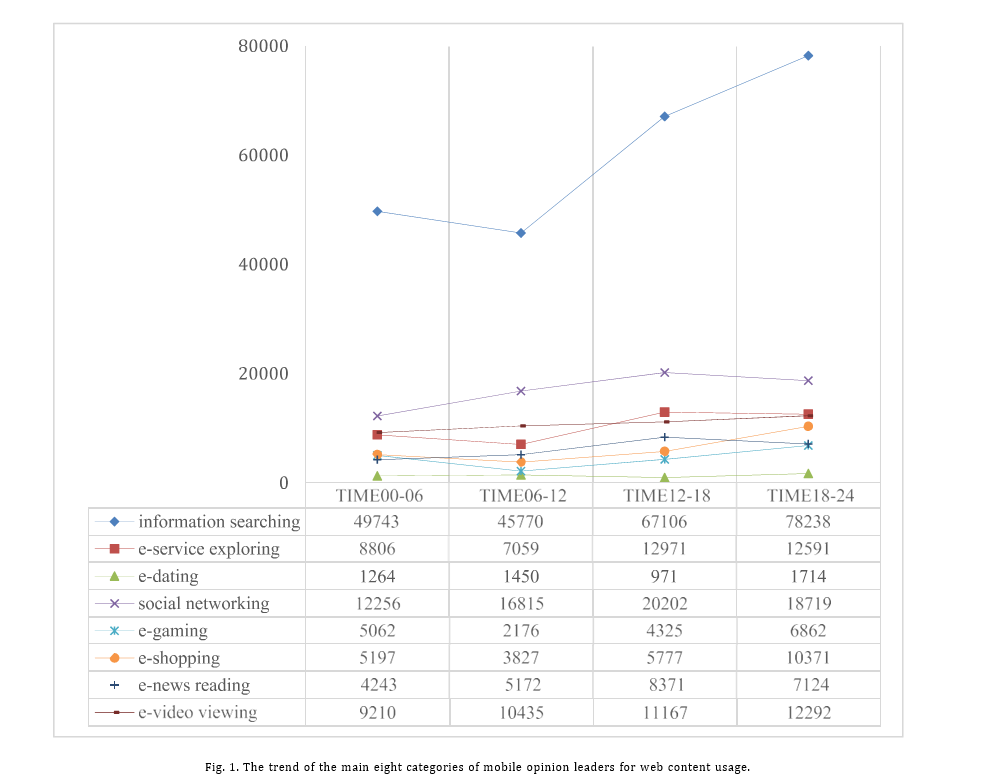
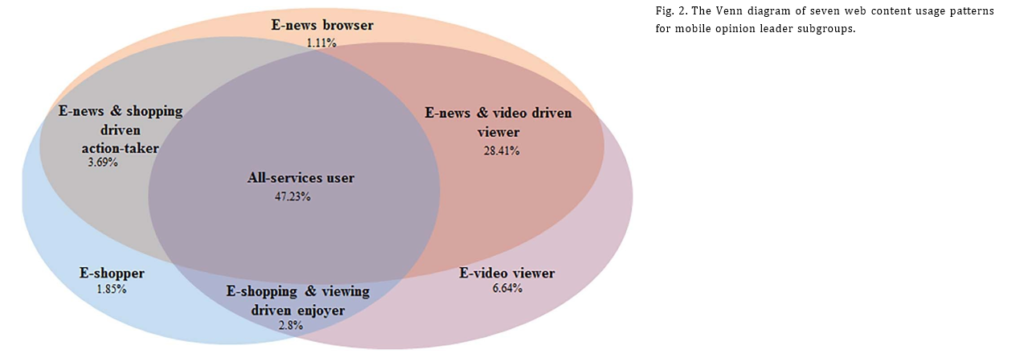
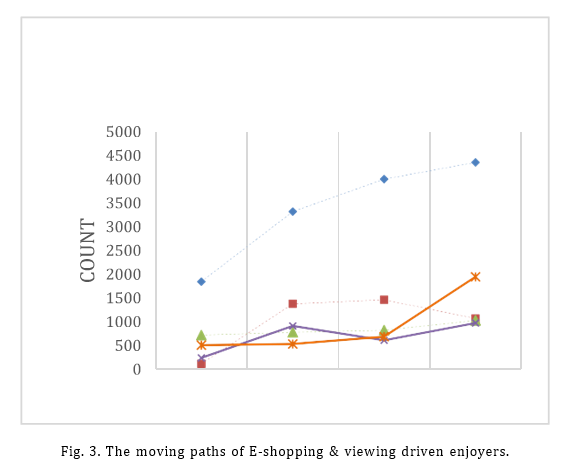
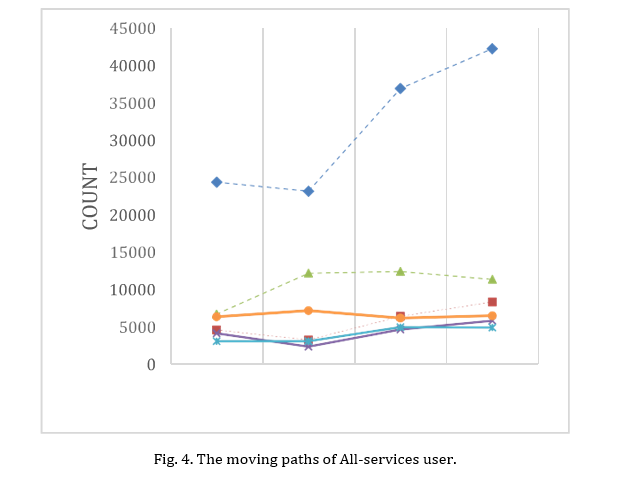